Data science is a team sport, and when you’re building and structuring your data science team, keep in mind that you’re running a marathon, not a sprint! It’s not an overnight process and takes time, experience, effort, and collaboration. In this blog, we’ll share our experience on how to structure a data science team, which we earned over the course of a decade.
Table of Contents
Stages of Data Maturity Model
To first understand how to structure a data science team for best outcomes, you need to first reflect on a critical aspect — your data science maturity. Are you at the data-awareness stage, or is data followed as a culture in your organization? To build a data science team and structuring it, depends on this crucial aspect.
For a complete rundown of data maturity, check out our blog on what is data maturity.
You can also download the whitepaper here that talks about the maturity stages along with some must-have job roles for data science teams.
There are different ways you can structure a data science team:
- Centralized
- Decentralized
- Hybrid
- Specialized hybrid model – The hub and spoke
Let’s talk about them one-by-one.
Centralized Structure of a Data Science Team
This model to structure a data science team takes the top-down approach. It starts with the leadership’s belief in data and its effectiveness.
The central data science team is often housed within IT and is driven by what can meet most of the needs of the organization.
Suppose there are multiple units in the organization (Sales, Finance, etc.). Data science teams try to understand what the needs of those units are and plan their priorities, whether it is hiring people or getting the required tools.
It is usually a good structure to adopt if you’re just starting out with a data science team.
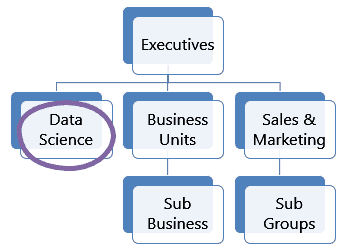
What Works
- As they are a central team, they can share knowledge and best practices. There is close collaboration between them.
- There is less redundancy, more efficiency.
- The best talent can be earmarked for the most important projects, as it is a shared talent pool.
- If it has the right executable leadership, they get the corporate priorities right and get funding and support from the executive.
Challenges
- If the central unit fails, it affects the entire data operation
- Business alignment sometimes suffers, particularly at the end-user level
- It is often seen as slow-moving, as each business unit has to submit requests to the central team, which goes through a process before the team picks it up from the queue
- If the central team doesn’t have adequate staff, they will have too much on their plate.
Decentralized Structure of a Data Science Team
Once the business units feel the need for data, this structure is usually used, usually in mid- to large organizations.
In this structure, the business units have their own data science teams, and these teams begin and scale-up in parallel. These teams align well with end-users, as they are housed within each business unit.
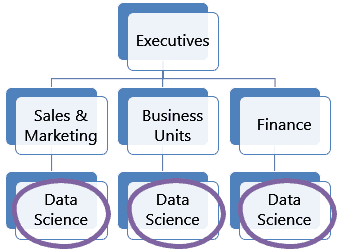
What Works
- They’re within the business unit and hence can work on that unit’s priorities.
- They can deliver results and quick wins, especially in the first few projects because they are aligned with the end-users
- Teams gain domain knowledge as they work within the unit
Challenges
- Talent and knowledge are in silos as there is no interaction between teams
- Different analyses by teams in each BU could lead to “conflicting truths.”
- When they strive to go beyond the BU to an organization level, teams often lack support from the executive
Hybrid Structure of a Data Science Team
This structure is a mixture of the above two formats. It balances control and efficiency.
There is a central data science team with a pool of talent, which is allocated into BUs depending on the requirements and priorities. Once the project is over, the team goes back to the pool and is earmarked for the next project. It consists of a typical matrix structure with dual reporting.
What Works
- It combines the best of both worlds. You can tailor and scale it based on organization size, know-how, and data maturity
- Talent understands the business as a whole due to the rotation into different units. They can see the outcome. They also become more competent with data
Challenges
Ambiguity in roles and ownership could take away the gains. They need to be defined clearly, and processes need to be in place.
The Hub and Spoke Model for a Data Science Team
This model consists of four components – the central hub, spokes, execution teams, and gray areas. Each component has a defined role. Let’s take a look:
Hub: Central group headed by a C-level analytics executive. Has the following responsibilities:
- Has hiring responsibility, getting people into the organization
- Sets up key processes
- Looks at the tools that can work
- Makes broader and universal decisions like data strategy
Spoke: Market-facing business unit to own and manage solutions. The responsibilities of the spoke are as follows.
- Deciding how to run the project
- How to interact with the end-users
- Driving adoption within the organization
- Tracking business benefits?
Gray area: Work with overlapping responsibilities, has room for maneuvering
Execution teams: Dynamic teams assembled from the hub, spokes and gray areas
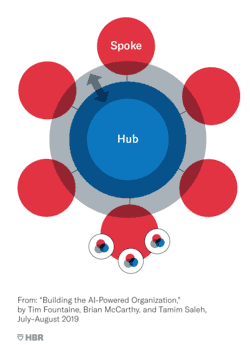
From the time an organization just gets started on data science to the time when it is running a hundred data science projects, the structure of data science teams keeps evolving. Let’s take a look at how the hub and spoke structure evolves.
Stages in the Hub and Spoke Journey
- Newborn: The hub is in charge of most decisions, as you’re setting up the practice for the very first time. Set up key processes. Business teams provide domain knowledge and managerial direction, but most of them come from the central hub.
- After 5-10 projects: You start transferring knowledge to the business units, and the hub begins shrinking. The spokes can make more decisions.
- Mature: The hub shrinks to a bare minimum. An analytics executive still leads it, and the hub still takes most of the strategic decisions. But the spokes make many of the execution and execution-level decisions. That way, the spokes grow in capability, and their teams have better domain knowledge.
We have a case study that you can refer to — the story of how Gramener organized its data science team over the years. We explained it in one of our webinars on building data science teams for completing projects successfully.
Check Out Our Data Advisory Workshop
We are covering everything we explained above in our official data science advisory workshop. The aim of this workshop is to help businesses assess their data maturity, create data science roadmaps, and build a strategy where they can quantify every single investment in their data science efforts.
Takeaways
We delved into the pros and cons of the centralized, decentralized, hybrid, and hub and spoke structures for your data science team. In most cases, we also saw that the hub and spoke model is the ideal method to structure your data science teams, as this gives much room for maneuvering. This could change depending on the way your organization is structured and your data maturity.
At Gramener, we work with top executives to help them transform into a data-driven organization. With our data science consulting and a variety of data advisory workshops, we lay a successful data science roadmap by assessing the level of data maturity of the organization. Take a free assessment and find out where does your organization stand in the levels of data maturity.