Innovations and technology trends eventually find a way to improve healthcare and medical practices. The applications and potential of Artificial Intelligence (AI) might be a game-changer for the healthcare industry. AI and its branches can help the medical & healthcare industry, from researching diseases to executing treatments. Natural language Processing (NLP) is one such branch of Artificial Intelligence that presents several different abilities. NLP use cases in healthcare have emerged as the key focus over the last few years.
By implementing NLP for pharma and life sciences challenges, we can transform different domains of the healthcare industry, such as helping discover new drugs, analyzing clinical research data, and understanding diseases. As such, NLP in healthcare is expected to grow to a 4.3B$ market size by 2026. This article covers how we can benefit from NLP in healthcare by covering the latest trends and use cases of NLP.
Table of Contents
What is Natural Language Processing (NLP)?
NLP is a sub-domain of the vast Artificial Intelligence field. As the name suggests, NLP aims to enable machines to understand natural language inputs. Interpreting voice inputs, understanding speech, or analyzing written or textual information are several examples of natural language processing.
How Does NLP work?
The working of NLP is quite similar to any other Artificial Intelligence model. Therefore, NLP requires a set of clean and pre-processed data organized in a way for an AI model to understand. This part of processing and analysis of data is known as Tokenization, and it includes dividing natural language inputs into tiny semantic units called tokens.
Such tokenized information allows machines to interpret any human language command by understanding the tokens. Moreover, there are two ways NLP models work:
- Rule-based decision-making: Rule-based decision-making is a conventional way of analyzing inputs against a fixed set of rules to derive a response.
- Machine Learning models: Machine Learning models are an intuitive way to implement NLP where statistical methods can derive model response accuracy over time through feedback loops.
NLP is now involved in many day-to-day applications in smart home voice assistants like Siri, Alexa, or Google Home. Another popular use of NLP in the business world includes the use of chatbots. Similarly, NLP can help utilize dormant unstructured data without tedious efforts. We will go through the significance and applications of NLP in healthcare below.
What is NLP in Healthcare?
In general, Natural Language Processing provides machines with the ability to search, process, and understand massive amounts of data. Notably, Big Data, Electronic Health Records, Telemedicine, and IoT applications have flooded the healthcare industry with colossal data sets. However, most of the available information is not put to good use. Almost 80% of medical data is unstructured; therefore, data like text, images, and signals are not helpful enough for machines to understand and research.
NLP in healthcare areas can address this challenge as machines can now access and use this mass amount of information. With NLP in healthcare, professionals can now utilize medical notes, pictures, and public information that has been available for a long time. It means that healthcare and medical companies can now understand the diseases, drugs, and patient responses to predict the best moves ahead.
Furthermore, it allows healthcare professionals to freely capture and manage unstructured data so that they can record it, share it, or process it as needed. NLP use cases can add up to better treatments, efficient medicines, better life quality, and long life expectancy.
Latest NLP Trends in Healthcare
NLP in healthcare is a buzzing concept for the industry as if it is to be the next disruptive technology. NLP use cases and abilities can be helpful in several different healthcare trends, as given below:
Better Decision-making for Healthcare Practices
NLP in healthcare facilities makes the information more accessible and understandable. Such thorough and useful information with AI and Big Data makes decision-making faster and more efficient for professionals. Through NLP, they can find the most-relevant treatments, medicines, and other care-taking factors at the tip of their finger. Therefore, NLP use cases lead healthcare to provide better treatments and avoid fatal errors.
Risk Assessment and Priority Patient Treatments
Late identification of a disease can cost us lives. As such, it is important to find the disease and assess its effect on the patient. The use of NLP and predictive analytics can make a difference as hospitals can deal with high-risk patients before it’s too late.
For example, the healthcare NLP algorithm was helpful in identifying at-risk Kawasaki patients with a specificity of 77.5% and sensitivity of 93.6% compared to a manual review. In such cases, NLP can identify which patients need immediate assistance and provide the right life-saving decisions.
Efficient Clinical Trials for Higher Success Rate
EHR, manual records, clinical trial data, and patient history are several ways to identify the effectiveness of a particular treatment on a unique patient. NLP can be useful as an analyzer to understand the drug, the procedure, and the patient’s conditions through unstructured data. Such matchmaking can substantially improve the treatment and make treatments more predictable.
Discoveries and Innovations in Medical Practices:
The growth and innovation in the healthcare domain have been gradual and at their own pace. NLP can expedite such a pace of medical discoveries. The existing materials about drugs, trials, diseases, and past successes can all help connect the dots. Such analysis can take due diligence of years for a manual research team. However, NLP can study this within much less time and present exceptional discoveries and new ideas.
These trends are backed by some acclaimed applications of NLP in healthcare, as given below.
Top 10 Use Cases of NLP in Healthcare
The Healthcare industry is a vast domain, including different aspects of drugs, clinical practices, patient data, etc. As such, NLP use cases in healthcare are of a wide range:
Data Redaction from Clinical Trial Documents
As covered above in trends, NLP is an excellent tool for processing many documents and unstructured information. However, there is another important application of NLP for data redaction from clinical trial documents. There are many data anonymization tools that help identify and redact patient’s personal information from clinical trial files and databases. Medical/health records consist of sensitive patient information and confidential details of the treatments and drugs.
With compliances like HIPAA and EMA, mismanaging sensitive medical information can lead to regulatory affairs or even legal actions. Therefore, redaction from medical records is extremely vital. NLP can help erase such sensitive information efficiently, protecting patients against threats like identity theft.
Pharma Literature Mining for Drug Development
Drug development is an integral part of the healthcare industry and is a time-consuming process. The healthcare industry can use NLP to analyze a variety of compounds and existing drugs to find insights for drug development. NLP can leverage all the unstructured text about the drugs from public sources, databases, and internal findings, providing actionable data for every stage of drug development.
Mapping Drug, Target, and Disease
This NLP use case is also about making the most out of vast information to derive concrete insights. For example, NLP can understand the drug’s formation and compound to understand how a medicine would react to a particular patient and disease. This mapping can make healthcare dramatically predictable.
Content and Context-based Entity Extraction
Entity extraction uses an application of NLP called Named Entity Recognition. It allows a user to extract relevant and valuable information from a data set. This application of NLP can be a crucial player during research or query resolution where a user is searching for a particular fact or answer.
Clinical Assertion Modeling and Diagnosis
Clinical Assertion modeling is an NLP use case that can ease the burden of medical professionals. This technique allows analysis of a patient’s history and appointment to find frequent symptoms to understand their condition better and provide the correct treatment.
Simplifying Clinical Documentation
NLP can help healthcare professionals with documentation needs to minimize their time on documentation and focus more on their crucial responsibilities. For example, NLP can summarize documents, find the key information, or convert an image into text (with Named Entity Recognition) to easily record electronically.
Computer-assisted Coding for Medical Billing
NLP in healthcare companies can help them with Computer-assisted coding (CAC) to extract billing details from a record and then translate them into standard billing codes. Using NLP for CAC eliminates the need for professional coders to perform the medical coding procedure.
Read More: Benefits and top 12 use cases of computer vision in healthcare
Using NLP for Medical Image Captioning
Captioning a medical image is among complex NLP use cases. This is an application where NLP is used along with Computer Vision. First, computer Vision would help comprehend a medical image like an X-ray scan and understand the picture frame. Then, NLP can caption that image with standard format and details such as problems, findings, indication, and severity.
Clinical Trial Matching with NLP
Clinical trials can be tricky to execute if tried manually. That is why NLP can be useful for accurate patient recruitment. Either NLP can extract the patient participation criteria and find a suitable subject from the database, or NLP can generate a list of questions for users interested in participating. For example, IBM reports that with their clinical trial matching solution, they were observing an 80% hike in enrollment.
Sentiment Analysis for Patient Experience
Patient experience is an essential part of the process for healthcare institutes. NLP can help analyze patients’ feedback and sentiments through unstructured data such as social media comments. Companies can also review the comments to find any red flags in their process compliances.
Gramener’s NLP-based Solutions for Pharma and Lifesciences
At Gramener, we help global pharmaceutical leaders decode unstructured text such as patient information and clinical trial data using natural language processing techniques. Let’s look at two of our live pharma and healthcare case studies.
Automated Masking of Sensitive Clinical Trial Data
A global healthcare company uses our automated solution to anonymize and redact patient healthcare information. The organization deals with clinical trial documents that run into thousands of pages and must be submitted to regulatory authorities such as the FDA. These documents cannot carry private patient information as part of regulatory compliance.
Earlier, they manually took up this complex and essential job of data redaction. But, no matter how proficient the person doing it, the process is time-consuming, expensive, and error-prone if you factor in the human fatigue that is a natural consequence of such a task.
Our AI solution deploys various named entity recognition techniques to handle Personally Identifiable Information (PII) and Protected Health Information (PHI) following an automated entity detection, extraction, relationship management, and anonymization process.
The benefit? The time taken to redact patient information has reduced from days to hours! It helps the clinical trial teams to submit documents to the regulatory bodies on time and without errors.
Extracting Information at Scale to Speed Up Clinical Trials
Clinical trials are conducted on humans to answer research questions and evaluate medical interventions. Conducting clinical trials is time-consuming and expensive, running into various phases. Clinical trial firms need to identify patients that fulfill all the study conditions.
Our client, a global pharmaceutical leader, required peering over 7 Mn documents to capture the many parameters specific to the trials it was conducting. Our solution automated this task by creating a metadata repository of the documents.
The solution uses text analysis to identify different elements within the document, aggregate metadata, and process them, so they are searchable. It has resulted in a significant reduction in the time and effort involved in doing it manually.
Bottomline
The core uses of NLP are currently focused on utilizing the plethora of data that is untapped in the healthcare industry. In addition to that, NLP in healthcare can offer other advantages like speech recognition, speech-to-text, healthcare voice assistance, and many other applications. It is evident that NLP use cases have promising value and might end up transforming healthcare practices as we know them in the next decade!
Contact us for custom built low code data and AI solutions for your business challenges and check out pharma and life sciences AI solutions built for our clients, including Fortune 500 companies. Book a free demo right now.
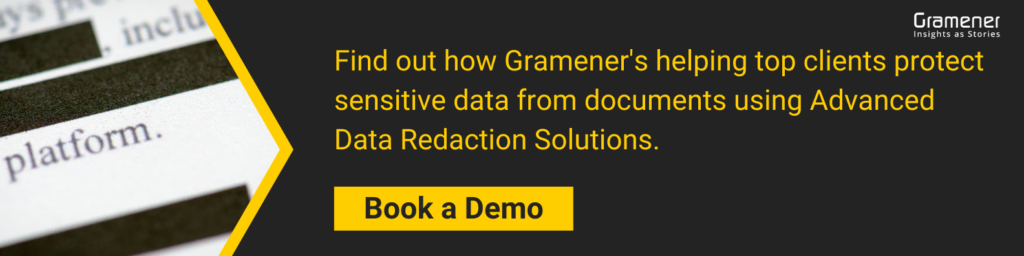