Artificial Intelligence (AI) and Machine Learning (ML) have fast-tracked the digital transformation of logistics and supply chains globally. There is a significant disruption in logistics, shortages in materials, skills, and labor, not to mention a rise in prices due to the COVID-19 pandemic and the war in Ukraine. This has forced companies to reimagine and reinvent the use cases for the supply chain with AI and ML.
Only the right combination of AI and supply chain can help companies tide over this crisis. To help you achieve this, you need to understand use cases and go through some well-established supply chain case studies. An in-depth reading of at least one modern supply chain case study may prepare you better to use AI and ML in the supply chain. Not to worry, you will find all you need in this blog.
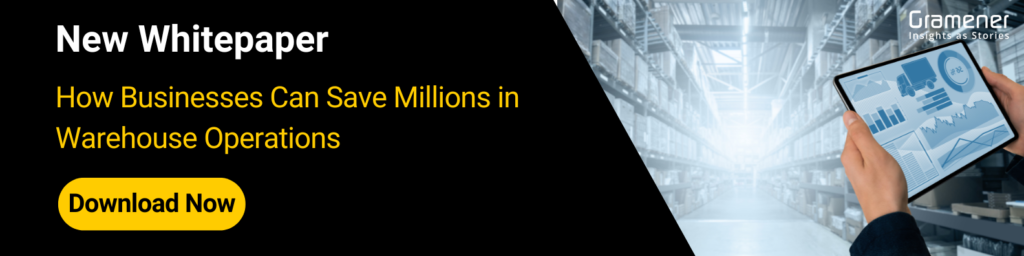
Table of Contents
Top 10 Use Cases of AI and ML in Supply Chain
Pre-tested and validated algorithm-based AI/ML models in the supply chain are already being used to analyze data and insights for:
Demand Forecasting
Demand patterns can fluctuate due to changing customer preferences, prices, and seasonal requirements. Predictive analytics of big data helps to predict demand accurately. Moreover, it makes sense to club it with capacity forecasting, and labor spending optimization.
Supply Forecasting
Supplier data on production and delivery is important to streamline activities. However, AI and ML in the supply chain can track metrics, develop benchmarks, and recommend vendor selection.
Inventory Planning
Both understocking and overstocking are serious threats to the business. For instance, if you go through companies using AI in supply chain case studies, you will find they manage to strike the right balance and shorten lead time.
Production Planning
Meeting the delivery date every time is a challenge for the production department. Digital resource mapping seen across multiple case studies in the supply chain ensures optimization. Digital twin technology is becoming popular to augment production performance, while computer vision defect detection is picking pace in manufacturing companies.
Warehouse Management
A warehouse operation collects a lot of data in a controlled environment. Simulations based on activity-level data reduce the manual measurements of product, layout, and labor effort to boost performance. Innovative applications like Intelligent Appointment Scheduler and Smart Task Allocation have been introduced to improve supply chain scheduling with AI.
Logistics Management
Traffic congestions pose a major threat to logistics. This hampers delivery timelines, which in turn results in hefty fines from clients to supply chain vendors. Route selection and scheduling can be optimized with the help of AI.
Pricing Management
Time-based pricing linked to market demands and competitor plans can help companies remain competitive. Thus, insights from AI in supply chain case studies analyze the impact and suggest dynamic pricing based on customer psychology, perceived value, and other factors.
Text Analytics
Web scraping, social media (SM) listening, and translation can help to track data from internal (shipment, supply, partner) and external (news, SM platforms, compliance) sources. AI and supply chain users, therefore, employ Natural Language Processing (NLP) techniques.
Customer Management
As automation, virtual assistance, and facial recognition technologies enhance customer experience, businesses need precise customer analytics. So, the use of AI in the supply chain is becoming necessary to increase customer engagement.
Artificial Intelligence collects real time data points and helps the business owners improve supply chain visibility to better manage their inventories, reduce delays, and offer better customer service.
Workforce Management
Apart from administrative functions, intelligent allocation of tasks through data analyses promotes AI and supply chain uses, as it operates at the intersection of data, people, and processes.
Challenges in the Logistics and Supply Chain Industry
Challenges are not new to the logistics and supply chain industry. Cost inefficiencies, technical downtimes, labor shortages, and bad customer experience can be disastrous for any business. However, the complexity and volatility across global supply chains present new problems every day. Innovative AI in supply chain use cases tackles these issues at the micro and macro levels.
Cost Inefficiencies
Cost inefficiencies are usually the result of inaccurate planning and inflexibility, which causes sluggish operations. Eventually, this leads to a drop in on-time in-full delivery performance, which spawns unhappy customers and falling revenues. Companies falter at this stage and adopt piecemeal approaches to solve specific problems like excess inventory, unavailability of raw materials, employee overtime, inferior quality, and so on. Whereas, saving both cost and time are a few benefits of using AI in the supply chain.
Technical Downtimes
Technical downtimes and breakdowns are caused by a lack of maintenance and poor equipment utilization, which can lead to an Operational Equipment Effectiveness problem (OEE). Add to this an unskilled, overworked, idle, or scarce workforce, and you have an Overall Labor Effectiveness (OLE) constraint as well.
Bad Customer Experiences
Bad customer experiences arise due to ignoring customers’ needs, failing to give quality customer service, lengthy delays, and company representatives who lack knowledge and etiquette. Cognitive and self-learning AI in supply chain use cases can prevent this by predicting what customers want, even before they realize they want it. A basic example is that of a chatbot that answers customers, instead of making them wait in queue for a call center agent.
Machine Learning in Supply Chain
With organizations driving revenues through AI implementation across the board, including the supply chain, there is more interest in AI in supply chain use cases. However, there is consensus that emerging and mature supply chain technology gives a competitive edge with a high ROI on investments guaranteed.
Predicting an increase in the adoption of digital supply chain technologies, as well as technologies that improve human decision-making, Gartner has said:
- By 2026, 75% of large enterprises will have adopted some form of intralogistics smart robots in their warehouse operations.
- By 2026, more than 75% of commercial supply chain management application vendors will deliver embedded advanced analytics, AI, and data science.
A subset of AI, ML represents automated learning of implicit properties or underlying rules of data. ML “trains’” or learns and refines an algorithm to perform a task better until the machine gains a new capability. Large amounts of data -including previously unseen datasets are processed for ‘“inference.”’ ML models trained to infer, are used to analyze trends, spot anomalies, and derive insights. Insights from the self-taught ML intelligence are used to make business decisions and even change strategies if the feedback warrants it. In fact, ML makes authoritative recommendations based on past, present, and future (predictive) data trends.
Machine learning is of use in all the ten AI in supply chain use cases cited at the beginning of this blog. However, the benefits of machine learning in the supply chain are likely to disrupt logistics too:
Automated Vehicles
Platooning of semi-automated trucks is poised to revolutionize logistics in limited geographical areas like mines, military bases, and warehouses. Solution for driver shortage, fuel consumption, and extreme weather conditions.
Collaborative Robots
Lightweight ML machines collaborate with humans to carry out repetitive, hazardous, and complex tasks, typically in warehouses. Picking, packaging, assembly, and quality control can be optimized, especially when combined with cameras.
Computer Vision
Visual sensors and cameras clubbed with ML data insights, algorithms, and deep learning neural networks, help computers “to see” and “convolute” images. Industry uses include inspection, classification, barcode and QR code reading, detection of defects, load monitoring, etc.
Machine Learning in Supply Chain Case Study
The historic mRNA Covid-19 vaccine developed by Pfizer and BioNTech, needed an ultra-low temperature supply chain and storage solution, for a product that was to be maintained at minus 75 degrees Celsius, to be delivered across small points of use across the world, from two manufacturing plants. Undoubtedly, this ML application stands out, as it was completed in record time at scale.
Pfizer was able to design special shippers enabled with GPS-powered data loggers, also equipped with reusable batteries (shipped back to reduce waste) which provided total visibility on the temperature, location, and status of every single dose. They are now working on these technologies for future frozen AI in supply chain use cases such as gene therapy.
Pfizer has won the Gartner Power of the Profession™ Supply Chain Awards 2022 for “achieving a positive, quantifiable impact on customer or patient loyalty through the supply chain.”
Benefits of Machine Learning in Supply Chain
- Predictive and prescriptive forecasting
- High end-to-end performance
- Zero-latency network efficiency
- Contextual intelligence for business
- Smart warehouse optimization
- Production planning improvements
- Accuracy in factory scheduling
- Total transparency and visibility
- Maintenance of physical assets
- Cost reduction
- Supplier quality management
- Risk mitigation
Which Companies are Using AI in Supply Chain?
Audi AG
The world’s leading producer of premium cars is using AI and ML in its supply chain to ensure sustainability. Moreover, it has introduced a sustainability rating with a Code of Conduct for Business Partners for suppliers and mandates that they should in turn ensure compliance by upstream partners.
Boeing
The world’s leading aerospace company uses AI solutions in its supply chain through a slew of digital service contracts and agreements with partners. This helps them promote operational efficiency and situational awareness in flight, use of a maintenance performance toolbox, and flight planning to optimize routes.
Bosch
The world’s leading engineering and technology company uses an AI analytics platform capable of reading terabytes of data in seconds and can achieve zero defects. They use AI to solve challenges in inventory management, demand forecasting, and optimization of packaging sizes.
Cat Digital
The Digital and tech arm of Caterpillar, a renowned name in the field of construction and mining equipment, gas engines, industrial gas turbines, and locomotives, is using the data from its products to help companies conduct predictive analytics.
Deutsche Bahn
Germany’s leading railway operator has launched many AI and ML projects to transform into a Digital Rail. Some of them are digital signaling, predictive maintenance of switches, and integrated command and control. However, It has achieved world-class procurement status and invests in digital start-ups globally.
How Gramener can Help With Its Data and AI Solutions for Supply Chain
At Gramener, we offer a wide gamut of AI solutions for the supply chain and logistics industry. Discover multiple supply chain data and AI use cases, case studies, and innovative solutions we developed for our clients.
Digital twins for supply chain process efficiency is our latest offering. Additionally, we have solutions for performance improvement, smart warehousing, defect detection, cargo delay reduction, downtime prevention, and supply chain visibility projects.
Contact us for custom built low code data and AI solutions for your business challenges and check out supply chain AI solutions built for our clients, including Fortune 500 companies. Book a free demo right now.
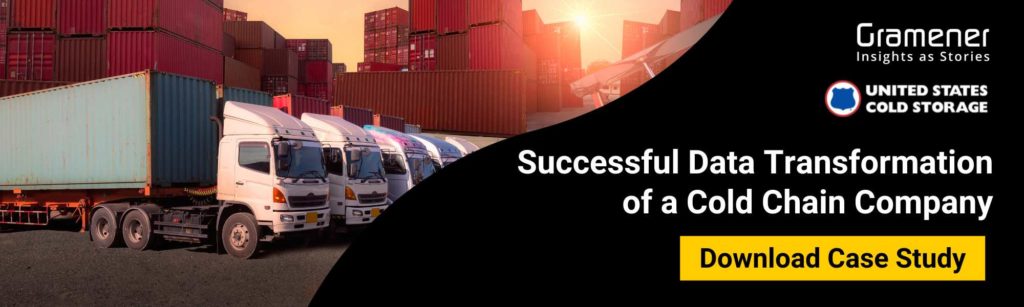