The concept of a digital twin is no longer novel today. It has evolved steadily in recent years to become a necessary tool for digital transformation for manufacturing companies. As enterprise digitalization is on the rise, predictive maintenance is also becoming popular. This article takes you through the role and benefits of a digital twin for predictive maintenance.
Table of Contents
The Need for Predictive Maintenance
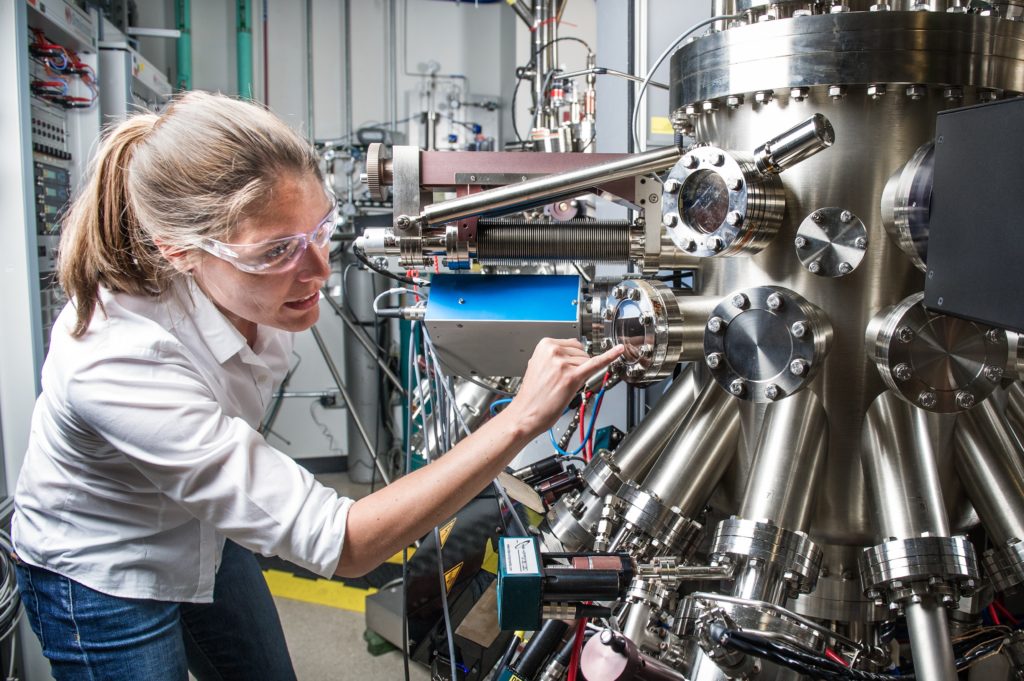
Here are the four reasons that make predictive maintenance essential.
Struggle to Attain Safety Compliance
As machines remain prone to damage and deterioration, frequent maintenance is essential. The frequency of maintenance will decide how long they will continue to function without breakdowns. Predictive maintenance is a proactive approach to the maintenance of equipment. It allows you to predict failure and track the equipment performance in real-time.
Preemptive Corrective Actions
This forecast helps in avoiding situations where a machine breakdown can lead to production downtime. The early analysis and detection of faults also help meet safety compliances and norms in an industrial environment. One of the vital aspects of predictive maintenance is condition monitoring.
It involves fetching real-time data through IIoT (Industrial Internet of Things). It is an essential aspect of the industry 4.0 revolution. The technology enables manufacturing companies to detect possible breakdowns and ensure continuity in operations.
Detection of Possible Future Errors
Predictive maintenance also helps in the early detection of problems that can snowball into bigger issues at a later stage. Manufacturing industry leaders can expect improved decision-making through real-time monitoring of equipment. The overall efficiency and lifespan of the machine will also improve.
A breakdown can lead to significant production losses for any manufacturing business. The business efficiency and growth rate of organizations also get affected. A few minor shortcomings can reduce the equipment life and lead to unexpected downtimes. It will lead to a situation where you will face recovering expenses, unsatisfactory production quality, and no equipment analysis.
Catching Shortcomings in Early Stages
When we talk of the fourth industrial revolution, it involves automating production processes through various technologies. Some of these include the Internet of Things, big data, and machine-to-machine communication methods. Predictive maintenance is a combination of all these elements.
Predictive maintenance involves data ingestion and its analysis through machine learning algorithms. It helps in extracting relevant insights and generating reports to understand the current state of the machine. There is also information about possible upcoming challenges. Timely detection of problems helps in preventing breakdowns that can affect the quality of production.
Reports also give a clear picture of the durability of a machine and its possible downtime. Your manufacturing plant can also plan for replacement machines in time to avoid business disruption. One of the reasons behind the improved success of predictive maintenance is the success experienced by manufacturing businesses. Predictive maintenance is a subset of predictive analytics in manufacturing. Predictive maintenance solutions are essential for manufacturers because they can help reduce production delays due to unplanned machine down time, improving the quality of manufactured goods, optimizing preventive or corrective actions taken on assets, and ultimately keeping business costs down.
Introducing Digital Twin for Predictive Maintenance
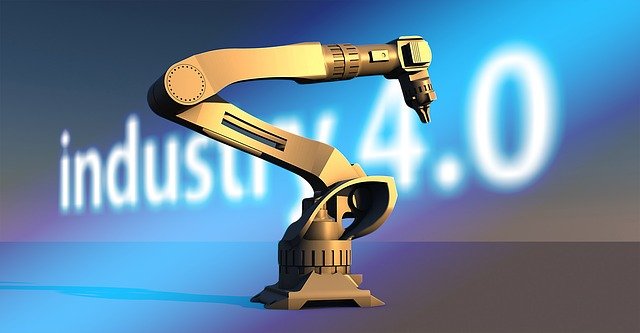
How does the digital twin process work?
The problems faced by any manufacturing business in an industrial environment can be far and wide. The industry 4.0 revolution has already shown that predictive maintenance can be a game-changer. Predictive maintenance through digital twin technology factors conditional monitoring, where data gets collected in real-time through sensors.
The health and working conditions of machines get monitored against historical data. It involves analyzing past failures and their criticality. The relevant data comes from the shop floor management systems of manufacturing units.
Neural networks help in detecting anomalies in the incoming sensor data. The predictive digital twin models generate a pattern, which helps in predicting failures. If the present configuration of a machine is likely to lead to a failure, technicians can prepare for that. Data-driven decision-making becomes possible through localizing the issue, understanding its criticality, and charting an action plan to mitigate the problem.
According to Gartner, 24% of organizations that implement IoT projects are using digital twins. Another 62% of them are in the process or plan to use digital twins. Manufacturing environments around the world stand to gain a lot through this disruptive technology.
Benefits of Using Digital Twin for Predictive Maintenance
- Helps in improving product design by detecting problematic areas that often cause breakdowns.
- Gives an idea of potential degradation of machinery through regular monitoring of equipment.
- Offers an ability to calculate maintenance related KPIs. It is possible by combining historical data related to risk factors, failures, and operating scenarios.
- Forecasts the performance of machines in different scenarios and how certain factors can affect them.
- Simulates the different maintenance situations to enable technicians to understand how a particular fix will affect the equipment by testing it on a physical twin.
Challenges Digital Twin Solve in Predictive Maintenance
Optimization of the Manufacturing Process
Businesses can expect better manufacturing efficiencies by overcoming siloed processes. It leads to better service delivery for improved business benefits.
Predicting the Quality of Product
Operators can analyze various components of a physical process to detect issues even before they occur. This step helps in identifying the quality of the product being manufactured.
Increased Labor Utilization
Virtual representations of physical objects can integrate key financial and operational data related to labor and raw material. Availability of data in real-time and advanced analytics aid better utilization of labor and raw material.
Increased Production Capacity
Businesses can test and validate products even before their existence. A digital replica of such products allows engineers to identify shortcomings and overcome them before they go into manufacturing. This enhancement helps in speeding up the production with quality assurance.
Reduced Maintenance Costs
A predictive maintenance model uses a just-in-time-replacement approach for replacing machinery components. Components get replaced only when they are close to failure. Additionally, there are no unscheduled maintenance and labor costs.
Increased Equipment Lifespan
The lifespan of any equipment or machine gets increased due to predictive maintenance models. Businesses can schedule the right maintenance programs at the right time to improve overall equipment health and lifespan.
These are some challenges that the digital twin is overcoming for the manufacturing industry. As operations become complex, the wear and tear of machines are bound to increase. A digital twin helps in transforming your business through optimized operations. Besides optimizing processes, digital twins in predictive maintenance can improve efficiencies, detect problems in time, and continue offering innovative insights.
A contextual model of your machinery through the digital twin in the manufacturing process can help you understand the root cause of the problem. There will also be insights on offer that enable predictive maintenance. These intelligent recommendations help in improving the lifespan of machines.
You can gain complete clarity about the present and future conditions of your machines. There will also be recommendations on how you can improve the performances. Digital twins are more than a mock-up of physical machines in your manufacturing units. They collate data from different areas like IoT sensors, IT and OT systems, and information from other third-party sources.
How to Build a Digital Twin for Predictive Maintenance?
Here are the three stages involved in the building of a digital twin for predictive maintenance.
Data Collection With Smart Sensors and IoT Devices
When we talk of any diagnostic or measurement device, sensors are a vital aspect. The sensors available on the hardware or software can collect the device telemetry. That information is collected to build a digital twin model of the physical machine. The data then gets compiled to generate actionable insights.
Digital twin models then showcase the present working condition of the physical machine. It allows modeling, monitoring, and managing machines from a remote location. You can also identify the time required for the maintenance of those machines. The regular intelligence data also helps you find an optimal time when you schedule the maintenance.
Analytics by Trained Machine Learning Models
Models, kernels, and analytics get built at this stage. Kernels have all the information related to elementary functional and mathematical formulas. Models are nothing but kernels that got trained to work on a particular asset. You can either create new kernels or use the existing ones to create a model that represents an individual asset.
Visualizations for Exploring Insights
Different types of digital twins are ideal for varying use case scenarios. Here are some of the insights on offer through digital twins in predictive maintenance.
- Allows technicians to monitor and get predictive maintenance insights. The equipment downtime gets reduced and also helps in the creation of service-based business models.
- Gives marketing and sales teams insights on customer preferences and product usage. They can strategize accordingly to improve revenue.
- Enables engineers and architects to enhance product versions for improved efficiencies.
- Gives management strategic insights and recommendations through analysis of operational data.
Our Digital Twin Solution for Predictive Batch Quality Management
To help you understand better, here is an example of our process monitor solution. It is for monitoring the quality levels through machine learning solutions.
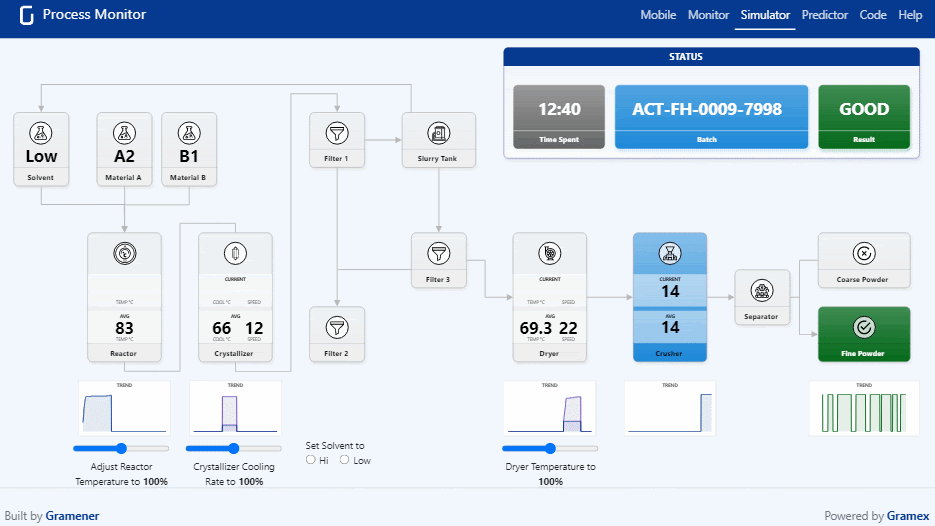
Here are various elements of the monitoring solution.
- Mobile alerts: Whenever there is any drop in quality, you can get notified even if you are on the go.
- Floor monitor: Operators can monitor the production process through large displays and get alerted when there is a drop in quality.
- Predict quality: You can understand the performance of the batch even without starting the production process.
- Simulator: You can improve the batch quality by running the previous batch with minimal changes.
Also Read: Optimizing pharmaceutical manufacturing with digital twin
Key Challenges to Build Digital Twin
Here are some challenges that you need to be wary of when building digital twins.
Getting Low Quality Data
Getting quality can be a concern. It will likely affect data-driven modeling and projections. Building accurate models are essential to derive meaningful insights. And that happens when you have quality data. Quality data related to operations is a lot more important for digital twins.
Data Communication and Latency
Communicating data in real-time is essential for building digital twins. It is because reflecting the accurate status of physical machines is necessary for digital twins to function successfully.
Focused Privacy and Data Security
Wherever there is data, concern about its security always remains. Several aspects need consideration, like how you will keep the data private and who gets access to it. Handling this sensitive aspect with utmost care is necessary.
Less Volume of Data
As the sensors will continuously monitor physical machines, they will produce a lot of data. Each device can generate a variety of data in varying formats. IoT devices collecting the data need to have the functionality to handle a large volume of data.
Advanced Visual Interaction
Some digital twins related to equipment inspection require visual interaction. You can use AR/VR interaction with physical machines. Interactions don’t need to only be with web applications, as scenarios can always differ.
Conclusion
Manufacturing businesses can enjoy a lot of advantages by opting for digital twins in predictive maintenance. Besides detecting problems in machines in their early stages, manufacturers can also improve the lifespan of their physical assets. There are also benefits related to labor costs and preventing unplanned maintenance.
Interested in learning more about how you can implement digital twins in your manufacturing business? Contact us today, and our experts will assist you right away.
Contact us for custom built low code data and AI solutions for your business challenges and check out digital twin solution built for our clients, including Fortune 500 companies. Book a free demo right now.
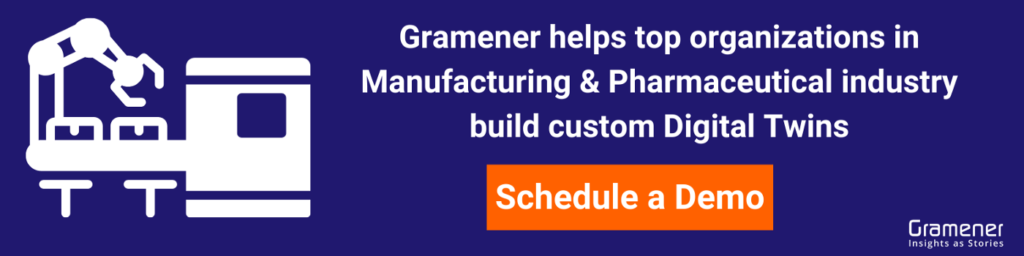