Have you ever watched an unboxing video?
Unboxing videos are a rage online due to the intrinsic desire to acquire products that satisfy our needs. The fact that these videos do not jump straight into a product review is testimony to the role packaging plays in a consumer’s mind.
In addition to aesthetics, packaging protects the product from damage and deterioration, informs consumers, and lets companies differentiate themselves from competitors. Therefore, defect detection is also an important aspect of packaging, as it ensures the product is in good condition and meets the expected quality standards. So, it is imperative for businesses to get their packaging and defect detection act together.
Unfortunately, packaging defects are widespread across industries leading to a loss in revenues, brand image, and product credibility. Add to this the pain of product recalls, switching loyalties of consumers, and product value miscommunication, and you have a marketing disaster on your hands.
Rewind to the beginning of the process, and you will realize that packaging is an integral part of supply chain management. Bad packaging impinges on distribution flow, product evaluation and categorization, customer experience, and the environment.
Table of Contents
Defect Detection in Packaging
At a micro-level, detecting packaging defects is part of product performance improvement. On inspection, defects that adversely affect quality are classified as:
Critical Defects
This means the packaging is unsafe for the user (damage to property, harm to life), and it fails the inspection as there is zero tolerance. For example, infestation with pests; growth of fungus, mold, and mildew; and sharp or flammable objects.
Major Defects
This interferes with the product’s function, appearance, and performance. If the defect numbers are high on inspection, the end-user is likely to return, demand a refund, or file a complaint. For example, torn or damaged packaging; warranty card or user manual missing; incorrect labeling and assortment.
Minor Defects
This includes non-conformance to specifications of all types, which may or may not catch the end user’s attention. On inspection, if the recurrences are higher than the high threshold level, we can not pass the order. For example, minor scratches, air bubbles in the glass, and illegible shipping marks.
While everyone follows the Acceptance Quality Limit defined by the International Organization for Standardization (ISO), we cannot ignore packaging regulations. They pertain to the thickness of packaging material, warnings that should go with polybags, and compliance with toxic material use guidelines. In addition, every industry and importer will have a customized quality checklist to guard against packaging defects.
At Gramener, we have a strong understanding of various defect detection techniques for different kinds of packaging across softline and hardline products, as well as electrical, electronic, and industrial components.
Discover how Gramener is revolutionizing industries with cutting-edge Industry 4.0 technologies in our latest video!
We can use a combination of prescriptive, predictive, and preventive analytics, computer vision, image processing, machine vision, and digital twin technologies to detect defects in packaging.
Learn more about our computer vision solutions.
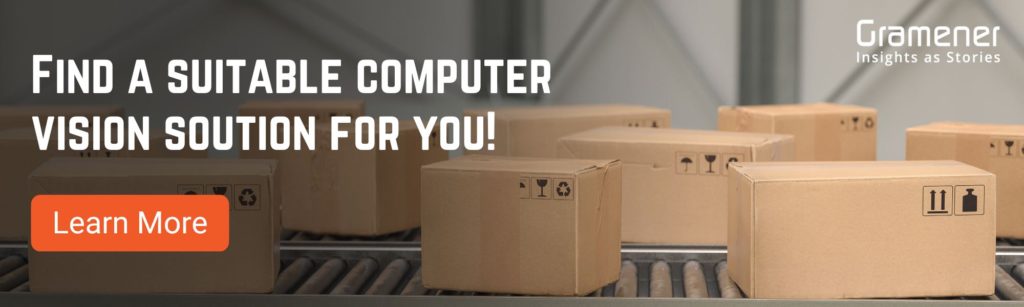
Detecting Packaging Defects in Manufacturing
Computer vision applications are useful for identifying and correcting mistakes in barcodes, product information, RFID tags, and asset IDs, and in meeting packaging standards. When combined with optical character recognition and augmented reality, or Artificial Intelligence, computer vision can even scan labels on packages that are being moved in warehouses.
Depending on the solution deployed, some defects can be rectified by alerting humans on phones or by an alarm on the dashboard. We can carry out some corrections through automated actions. Regular inspection reports on all these packaging features can help to optimize labeling and boost traceability in supply chain management.
Gramener has helped global companies identify their supply chain bottlenecks and design optimum workflows after identifying process and product defects.
Packaging Defect Detection for Perishable Goods
Quality assurance is of prime importance when it comes to perishables. Sorting and grading dairy products, meats, seafood, fish, and fresh produce helps to determine what bins (ripe, unripe, rotting, damaged) they go into.
Computer vision techniques help to grade perishable goods through a no-touch process. The analysis of, say, fruit images can help determine the size, shape, color, blemishes, and texture of fruits. It can further determine the specialized packaging requirements to prevent degradation.
Both refrigerated and non-refrigerated produce need preparation before packaging and handling. It includes pre-cooling, air-tight wrapping, foam inserts, padding, bubble wraps, cold packs or dry ice, and leak-proof box liners.
Common packaging defects of perishables include:
- Over-stuffing, making chemicals from packaging flow into the food
- Incorrect use of breathable and vacuum bags
- Exposure to temperature fluctuations
- Damage due to contaminants
- Wrong choice of packaging material
Gramener’s supply chain management solution experts ensure the farm-to-fork safe delivery of perishable goods with the help of the latest technologies.
Read More: Computer Vision AI is Unbeatable for Defect Detection in Manufacturing
Gramener’s Defect Detection Capabilities for Pharmaceutical Packaging
Our computer vision-based recommendations have helped pharmaceutical majors identify and fix defects during the pharma manufacturing process, guiding packaging.
Here is how it works:
Using sophisticated computer vision technology and allied tools, Gramener helped a global pharma firm detect anomalies while manufacturing tablets. For instance, we can trace capping, chipping, and discoloration, to faulty formulation, erroneous machine setting, and incorrect mixing of dye and binder.
Computer vision can detect wrong pill positions, tablet counts, inferior drug quality, slow production speed, etc., thus preventing the packaging of defective medicines and improving production performance. Product and process defects, when identified during manufacturing, also guide packaging optimization.
Packaging Optimization with Computer Vision
Manual inspection for packaging defect detection is time-consuming and tiring. AI-based computer vision models are trained to see, analyze, and interpret millions of images, visual data, and videos. A trained machine learning algorithm can detect defects in split seconds after processing an extensive data set of visuals. It can also ‘predict’ a defect before it becomes apparent to the human eye.
Computer vision models detect the defects in the following way:
- Object/Defect Classification: A defect in the blister pack is classified as a ‘tear’
- Object/Defect Recognition: The tear is labeled as a defect
- Object/Defect Localization: The location of the tear is marked with a bounding box
- Object/Defect Identification: The tear on the blister pack is identified with a bounding box and assigned a label
- Object/Defect Verification: The tear is verified to ascertain if it is a tear or a loose seal
- Object/Defect Segmentation: The pixels in which the tear is detected are shown as a segment map
Deep Learning, a machine learning method, uses a layered algorithm structure that imitates the brain to help detect defects. We call this structure of algorithms the Convolutional Neural Network (CNN), and it can function without human intervention. We can build computer vision models around many algorithms devised to solve different problems. Region-based CNN, Fast R-CNN, and Faster R-CNN are all algorithms that focus on specific regions in an image to identify an object.
However, the real-time object detection algorithm, YOLO (You Only Look Once), is popular. It is based on transfer learning which is considered far more ‘intelligent’ and detects defects with high accuracy and speed.
Conclusion
Gramener’s domain expertise can help identify the root cause of these defects. Whether those defects are traced to the sourcing and suitability of packaging material, handling of packages, transportation, or storage, we can find a fix. Looking for a custom defect detection solution for your assembly line?
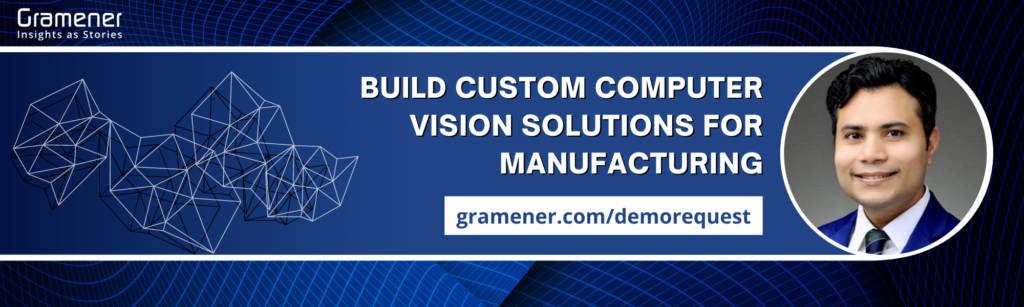