This article focuses on how organizations can transcend to higher levels of data science maturity. Here, we’ll talk about 5 levels of data science maturity, a free maturity assessment framework, and dimensions of data maturity to build a robust roadmap.
Find out where does your organization stand in the levels of data science maturity
Take Free Data Maturity Assessment
Table of Contents
What is Data Science Maturity?
Data science maturity is the measure of how well a company utilizes the data it produces. The more efficient an organization is in this task, the higher is its data maturity. So, organizations can treat data maturity as a compass on their data journey – to help them find where they are and make sure they are traveling in the right direction.
The journey towards becoming a data-driven organization could take years. Following data maturity can help you discover if you are going on the wrong path sooner.
The question is, how do you assess it?
For this, we need to understand the five levels of data science maturity based on Gartner’s data and analytics maturity model.
The Five Levels of Data Science Maturity
👉 The first level is all about the early stage of approaching data. The organizations at this level of data maturity use data only when they need it. They do not generally put their trust in numbers, and data adoption lacks leadership support.
👉 The second level has organizations that have had some early taste of success with data. Individual business units pursue their data initiatives. While there are some attempts to formalize some parts of the process, there is an utter lack of standardization.
👉 The third level is where an organization develops a clear vision for its data future, and standardization starts to set in, thanks to leadership backing.
👉 At the fourth level, data leadership roles like CDO emerge. There is a very clear linkage of data science with ROI for all the initiatives that the organization undertakes.
👉 At level five is where an organization becomes a data leader in their industry.
There is a clear demarcation between the organizational approach between the first two levels and the last three. In the effort to move up to the higher levels of data maturity, an organization must put data at the center of its business strategy.
As surprising as it sounds, the fact is that most of the organizations in the industry are pretty far into the first two levels. According to Gartner, they found out that 87% of organizations are at low maturity levels.
Why Do Organizations Get Stuck at the Early Levels?
Very few organizations are data-driven or data leaders. Many organizations get stuck at the first two levels with the challenges they face with respect to quantifying their goals, funding their data initiatives, honing people skills, influencing cultural change, implementing data science strategy, and enhancing data literacy. The question is how to navigate these challenges?
Data maturity is the starting point. Therefore, we must begin by assessing where we stand. To assess data maturity, one needs to answer a series of questions. They cover different aspects and disciplines within data across the different stages of the data life cycle.
At Gramener, we’ve come up with our own research and methodology and put together a data maturity assessment framework.
Take our 5-minute assessment and get your organization’s FREE data maturity report.
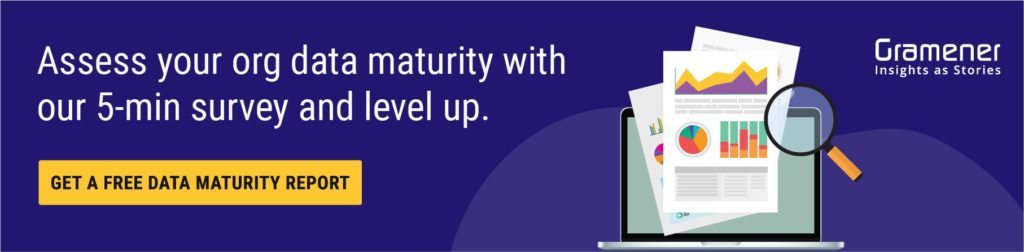
What Aspects Must Organizations Consider When Embarking on Data Maturity?
There are five dimensions of data maturity – vision, planning, execution, value realization, and data culture.
Data native organizations define their business vision, plan the data science goals and execute them efficiently. They can accurately quantify the value of their data science projects and propagate data as a culture. These five dimensions can be improved by focusing on 12 elements or activities as given below –
So, if you want to improve the vision, you work on clarity and strategy alignment. If you want to improve execution, you should work on the roles, skills, data analytics, data consumption, and data engineering processes.
With our data maturity assessment, you can ask yourselves similar questions. Your score for each of these elements will contribute to the overall score. It would help you find out your organization’s data science weaknesses and priorities.
How Does the Data Maturity Assessment Help an Organization?
Data maturity assessment provides an organization with a maturity score. For example, you can be at level two, with a 2.02 on the scale. In terms of dimensions, an organization may score higher in some dimensions and lower in the rest. Thereafter, we would look at the elements and their respective scores. It will clearly demonstrate your priority areas based on the score as well as the importance of that element for your organization. For an element, if your priority is high and your score is low – it is an area of concern. If not, you could defer and work on it later.
The third aspect of the data maturity assessment is the recommendations – the short-term, mid-term, and long-term actions in each of the five dimensions. The recommendations are provided based on the organization and its priorities.
What Are the Business Benefits of This Approach?
Can improvement in data maturity lead to top line and bottom line improvement? How can you quantify and justify your efforts?
The International Institute of Analytics did an interesting study. They looked at 72 key metrics like, the revenue growth, revenue, stock price, etc of some organizations. They also looked at the analytics maturity of these companies. Upon doing the association and correlation analysis, they found a strong association for 59 out of 72 factors. Most of these factors go up when an organization’s metric goes up.
Similarly, Deloitte also observed that when organizations reach a higher level of maturity, they significantly exceed their business goals compared to their peer group.
How to Move Up the Data Science Maturity Levels?
Gramener’s data-to-value RADAR framework can help you combine the power of data maturity assessment with your own efforts in moving up the ladder.
Reflect
Data maturity assessment helps you reflect and look at the big picture. The next step is to reflect on the organizational strategy. Understand your corporate vision and the use of data to support that vision. This will help you come up with your data strategy.
Align
Combining the data maturity assessment with your reflection will help you align your vision with your efforts. Then you can pick projects that help you meet the business priorities.
To choose the strategic projects, you can brainstorm and identify a long list of key programs and then apply the levers such as impact, feasibility, and urgency to prioritize and come up with the quick wins and the strategic projects. Once you have prioritized, step two is to build a data road map. The roadmap will cover your data initiatives. For instance, strengthening the delivery process or improving the career path of your data science team, etc.
Define
Finally, how do you execute this? You have to define the people, processes, and technology that are required to execute your projects. After this, you define the delivery framework. For instance, how do you manage the project life cycle? How does it integrate and improve collaboration with the rest of the teams like the business team, technology team, and the other stakeholders in the process? Do you have the skill sets within the house? How do you execute your projects?
Adopt
Once you execute the project, you need to make sure that the end-users come in and embrace the solution. This is harder than it sounds. Data literacy is low in most organizations. You have to make people comfortable with data. But it is critical as it can be the insurance policy that ensures the success of your data initiatives.
Radiate
The next step is to measure the ROI by quantifying the business benefits from a data science project. Only once you quantify will you be able to move to the final step of radiating your initiatives and moving them to production.
For instance, how do you take your learnings to new teams? How do you manage change within the organization, and how do you build a culture of data-driven decision-making? Your project doesn’t end after successful delivery, you need to see how you can radiate and spread this message within the organization.
These five steps are what we call RADAR. If you pick the first letter of each of these steps, it pumps the word RADAR, and that’s the data to value methodology we follow. It is a cyclical process. Once you do one cycle of RADAR, it leads to the second cycle where you go back to the drawing board and start reflecting again. With multiple cycles, you can move up the ladder of data science maturity levels.
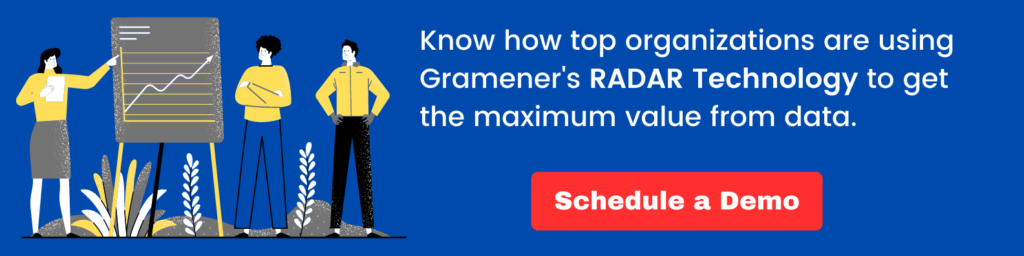